AI-based improvement of the evaluation of side view sonar images using deep learning methods
This dissertation deals with how deep learning models for the evaluation of side view sonar images can be successfully trained even with little training data. Among other things, a method for generating synthetic sonar images is presented and it is shown that enriching the training data set with these images can significantly increase the classification performance of neural networks.
We asked Yannik about his dissertation:
In what context was your dissertation written? Which projects or other factors particularly influenced your dissertation?
My dissertation contains research results that I developed at the DLR Institute for the Protection of Maritime Infrastructures (DLR-MI). The work began through a collaboration with ATLAS Elektronik GmbH, which commissioned DLR-MI to work on a project to analyse sonar images using deep learning methods. Dieter Kraus from the Institute for Water Sound, Sonar Technology and Signal Theory at Bremen University of Applied Sciences, the cooperation with the University of Wuppertal and ultimately the TMDT came about. Despite the number of partners involved, I am glad that I was able to research my topic very freely at DLR-MI.
What contribution does your work make to the field of research?
In the "sonar" domain, there is no large, publicly accessible data set like in other computer vision areas. I am therefore pleased that the method developed in my work, which is based on transfer learning, can be used to successfully train a Generative Adversarial Network (GAN) even with little data and that the images generated in this way are helpful in improving the classification performance of neural networks. In addition, my work is the first to use transformers for the detection of objects in side view sonar images, which show better detection performance than conventional digital image processing methods.
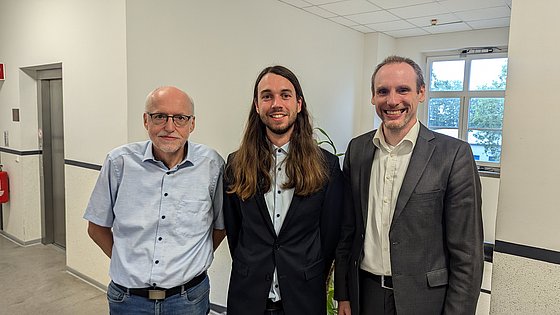
What's next for you and the topic?
It's more or less the same as before. I will continue to research the use of deep learning methods for analysing sonar data at DLR-MI. The application of algorithms on-board an autonomous underwater vehicle, for example, is a key point in giving such systems more intelligence. Automatically recognising what lies on the seabed is relevant for the removal of contaminated sites from the North and Baltic Seas, among other things. Our aim at DLR-MI will also be to build up a larger team that deals with the processing and analysis of hydroacoustic data.