AI-based improvement of the evaluation of side view sonar images using deep learning methods
The dissertation by Dr.-Ing. Richard Meyes investigates the transparency and interpretability of learned knowledge representations in artificial neural networks. The focus of the study is on the application of neuron ablation, a method known from the neurosciences, whose transfer to the research field of artificial intelligence is being investigated.
We asked Richard about his dissertation:
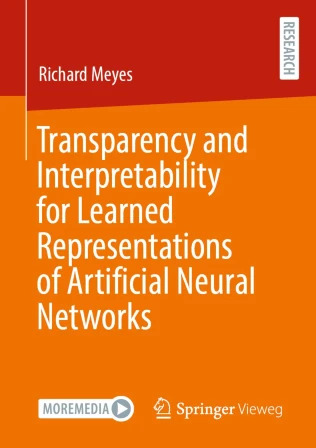
In what context was your dissertation written? Which projects or other factors particularly influenced your dissertation?
The idea for the dissertation topic was strongly influenced by my time as a research assistant at the Institute of Neuroscience and Medicine at Forschungszentrum Jülich from 2013-2015. During this time, I researched how the brain processes visual information that we perceive with our eyes every day. In particular, I focused on the interplay of neurons in the visual cortex and how this interplay is synchronized with the so-called saccadic eye movements that are characteristic of primates.
After my time at the research center, I worked as a doctoral student at the Institute for Information Management in Mechanical Engineering at the Faculty of Mechanical Engineering at RWTH Aachen University and found my way into engineering sciences with points of contact to German industry and business. Against this background, I no longer dealt with biological systems but with machine learning systems. Since then, I have been working in the research field of artificial intelligence and have brought the two research fields of neuroscience and artificial intelligence together to a certain extent in my dissertation.
What contribution does your work make to the field of research?
Probably the biggest contribution of the thesis is the publication of the first paper of the dissertation entitled “Ablation Studies in Artificial Neural Networks”, which has been cited about 275 times to date and has laid the methodological foundation for all further work of the dissertation in 2019. In the thesis, I applied the principle of neuroscience-inspired ablations to individual neurons and groups of neurons in artificial neural networks for the first time and was able to show that neurons can be classified as contributing to a learned task based on their specifically trained functions.
Building on this methodological contribution, I then investigated a number of different neural networks in their application in the industrial sector and was able to demonstrate, for example, which parts of an artificial neural network are responsible for recognizing individual handwritten numbers, for controlling an actuator to balance a pendulum rod or for detecting cracks in sensor data from a deep-drawing tool for the production of car body parts. In this way, I was able to make transparent which task individual neurons and entire sub-areas of an artificial neural network have for a learned task.
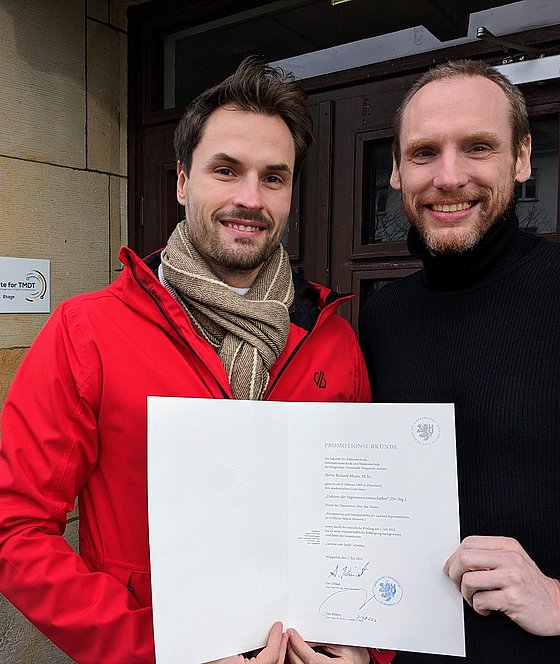
What's next for you and the topic?
The topic of transparency and interpretability of artificial neural networks continues to be of great interest for applications in the industrial environment. For engineers in particular, who are used to full transparency regarding the methods and processes used, it is crucial to be able to trust an AI that operates in productive value creation operations. The topic is therefore a permanent focal point in the projects of our “Industrial Deep Learning” research area and accompanies us more or less focused in everyday life.