Welcome to the TMDT Hall of Fame!
Here we present TMDT's successfully completed dissertations. Learn more about the people and the research behind these accomplishments.
2024
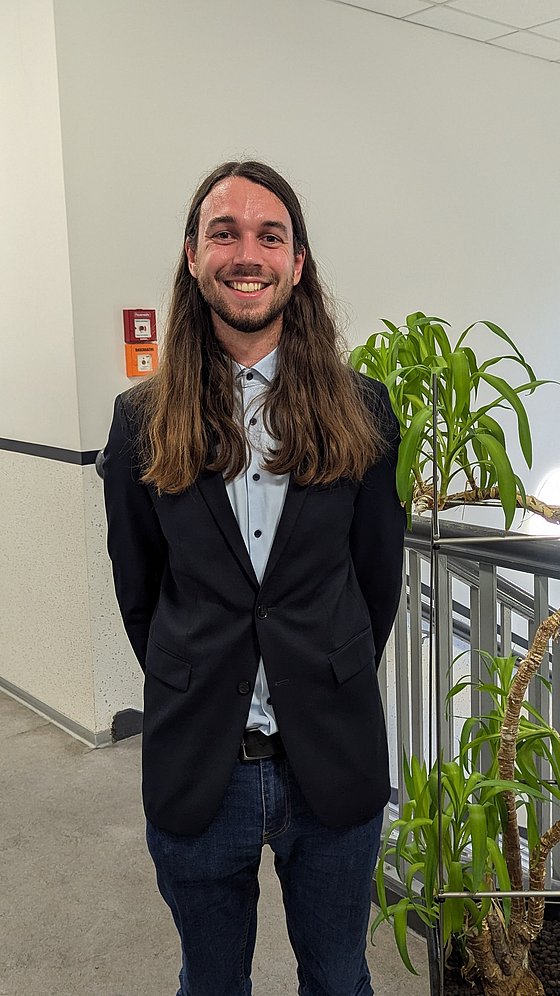
AI-based improvement of the evaluation of side view sonar images using deep learning methods
The dissertation by Dr Yannik Steiniger deals with how deep learning models for the evaluation of side view sonar images can be successfully trained even with little training data. Among other things, a method for generating synthetic sonar images is presented and it is shown that enriching the training data set with these images can significantly increase the classification performance of neural networks.
For more information click here.
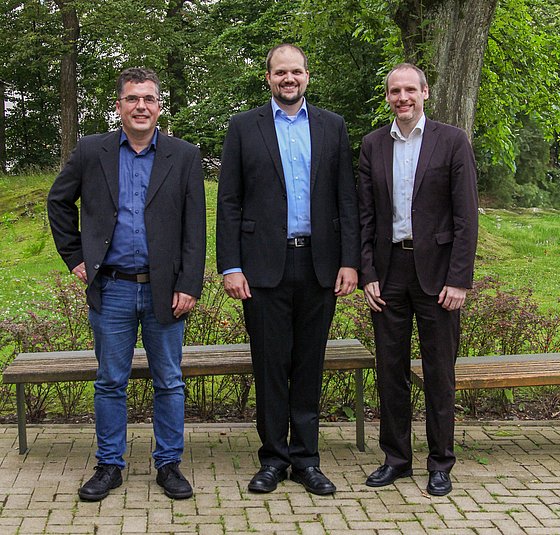
Semi-automated Refinement of Semantic Models
The dissertation by Dr Alexander Paulus deals with the annotation of data sets using semantic technologies. He describes a new approach focussing on humans in the annotation process and presents support systems based on machine learning methods.
For more information click here.
2023
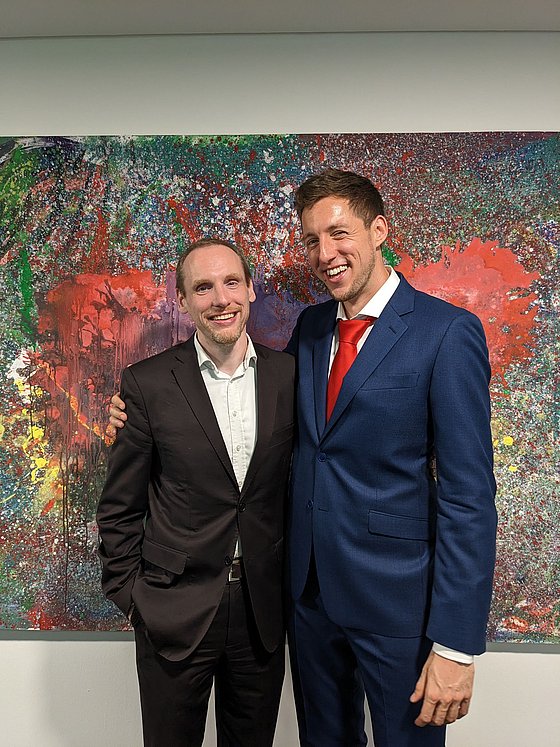
Digital shadow for urban freight traffic management
The dissertation by Dr Thomas Otte deals with freight transport in urban areas. In particular, it presents an approach for the systematic acquisition and utilisation of data on the status and changes in the status of the urban freight transport system, which can be used by local administrations to control this system in a targeted manner on the basis of objective and quantitative data.
For more information click here.
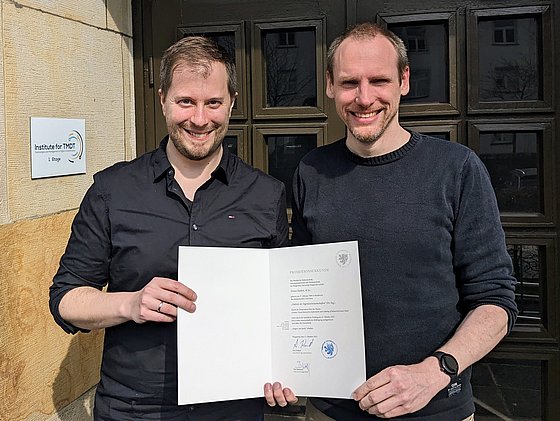
Guided Visual Interactive Exploration and Labeling of Industrial Sensor Data, Tristan Funken, 2023
Tristan's dissertation deals with an approach for guided exploration and labeling of industrial sensor data. Using real manufacturing processes, it is shown how this approach increases efficiency in the creation of high-quality labeled data sets and thus accelerates the use of AI models for the manufacturing industry.
Click here for further information.
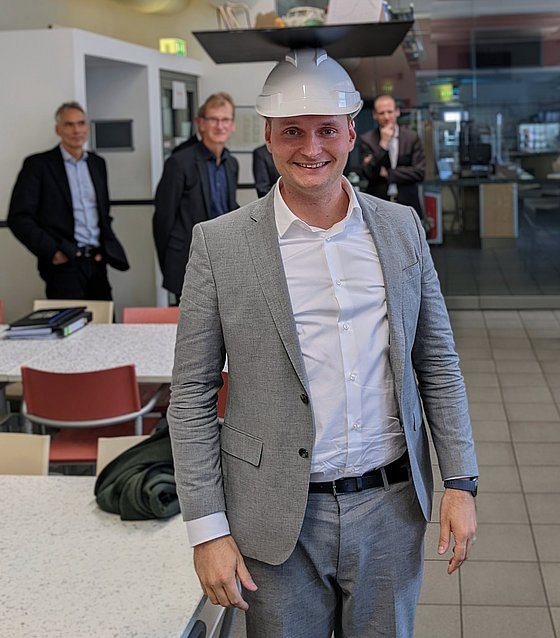
Predicting project progress as a contribution to virtual validation in the automotive sector, Oliver Böhme, 2023
Dr. Oliver Böhme's dissertation enriches the research field of virtual validation and project management in the automotive sector. It shows that predictive analytics and transformer models can uncover hidden patterns in project development. This increases transparency, control and cost efficiency by enabling project risks to be identified at an early stage and strategies to be developed.
Für mehr Informationen klicken Sie hier.
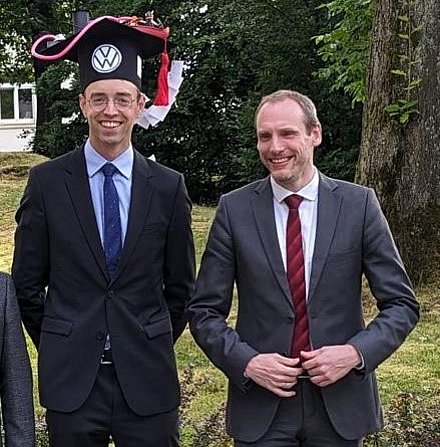
A Data-Driven Fleet Service: State of Health Forecasting of Lithium-Ion Batteries, Friedrich von Bülow, 2023
This dissertation examines machine learning models for predicting the state of health (SOH) of lithium-ion batteries. The focus is on the application in the practical context of battery systems in battery electric vehicles (BEVs) using fleet operational data.
For more information click here.
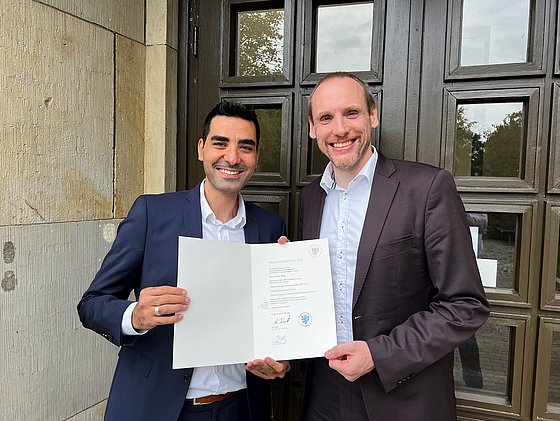
Machine Learning-based Predictive Quality in Manufacturing Processes, Hasan Tercan, 2023
Hasan Tercan's dissertation investigates quality predictions in manufacturing processes using machine learning. He introduces a process model called MERLIN, which is complemented by methods such as transfer learning and continuous training to increase the data efficiency of the learning models.
Click here for further information.
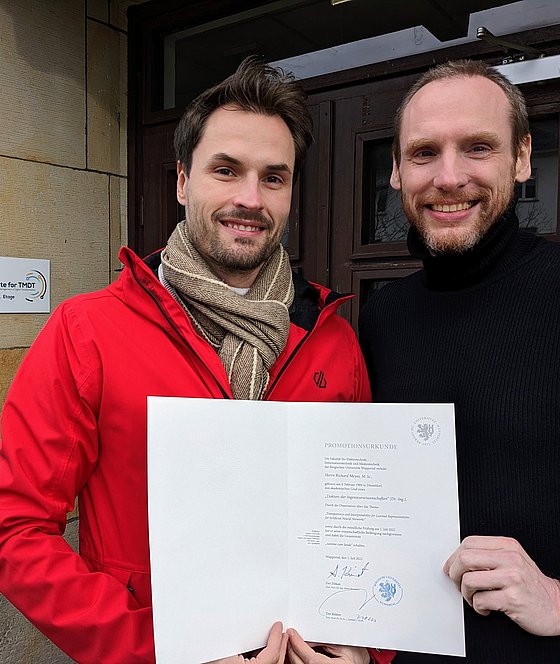
Transparency and interpretability in artificial neural networks, Richard Meyes, 2023
The dissertation by Dr Richard Meyes investigates the transparency and interpretability of learned knowledge representations in artificial neural networks. The focus of the study is on the application of neuron ablation, a method known from the neurosciences, whose transfer to the research field of artificial intelligence is being investigated.
For more information click here
2022
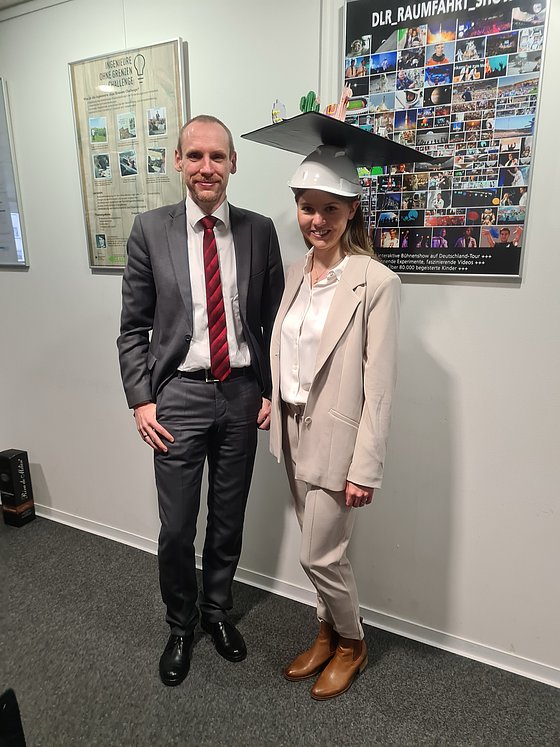
The dissertation of Dr Schirin Bär deals with the development of a reactive job shop scheduling solution for flexible manufacturing systems. Reinforcement learning strategies are shown to train cooperating agents that are generalised to handle unknown jobs and situations.
For more information click here.