GenISys
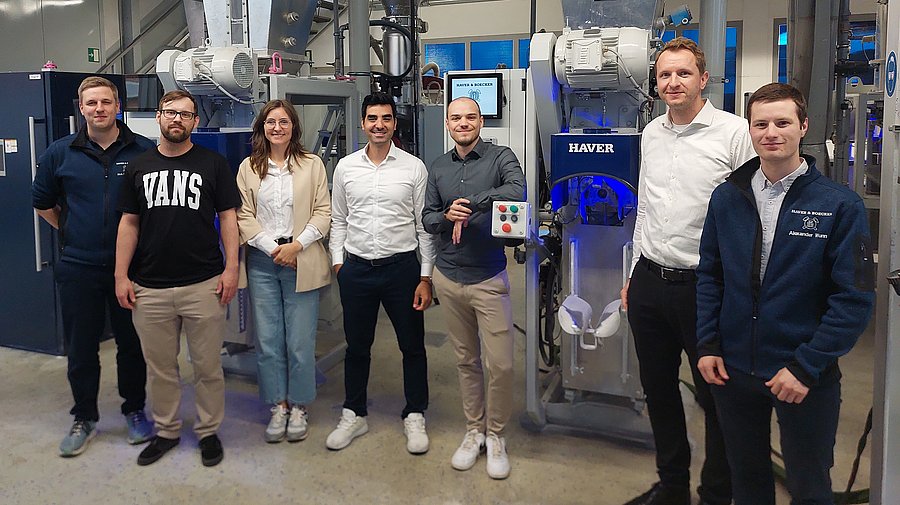
The team behind the GenISys project (from left to right): Dirk Bergmann (Haver & Boecker OHG), Kai Tybussek and Corinna Weber (SNAP GmbH), Dr. Ing. Hasan Tercan and Antonin Königsfeld (TMDT), Dr. Daniel Kliewe and Alexander Wunn (Haver & Boecker OHG)
Intelligent system for resource-saving plant configuration with generative AI technology - GenISys
Automatic filling systems are often used for the large-scale production of powdery or granular products such as cement and building materials. However, the configuration and parameterization of these filling systems is still a complex and resource-intensive process. The current configuration process begins with laboratory testing of the product, including the determination of key material properties such as density, particle size, abrasiveness and moisture. This is followed by an iterative prototyping phase in which the system components are manually selected for the specific product and parameterized through empirical experiments.
However, conventional configuration methods lack scalability and agility for various reasons. On the one hand, configuration is complex and resource-intensive, as the manual reparameterization of filling systems leads to longer lead times and costs; on the other hand, operation depends largely on the experience of the operating personnel, which does not scale with increasing process complexity.
Against this backdrop, the aim of the GenISys research project is to innovate the configuration and operation of existing modern filling systems by using digital technologies and generative AI processes. With the help of these methods, it should be possible to generate configurations without the need for extensive simulations, especially if varying material properties are to be taken into account. The trained models will be able to use data from the laboratory tests of the materials to be filled to generate a configuration that requires only minor adjustments by the operating personnel for an optimal filling process. By means of continuous learning, these models will be able to adapt to the conditions of the process and continue to learn. Together with the project partners, demonstrators will be developed and ultimately adopted as software and hardware solutions in the real process of filling line configuration in order to conserve resources and benefit from human-in-the-loop.
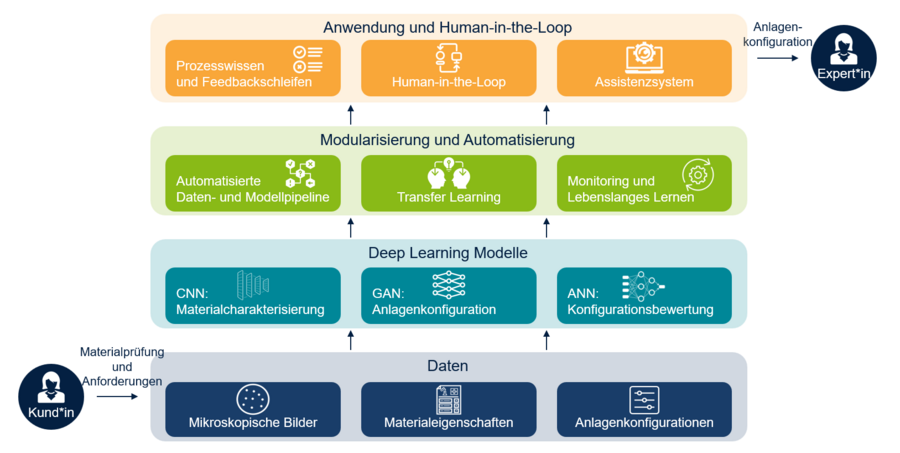