Industrial Deep Learning
In the field of "Industrial Deep Learning", we research deep learning technologies for industrial applications in order to realise innovative solutions in production, logistics and the environment. We combine basic AI research with industrial practice and focus on three main areas
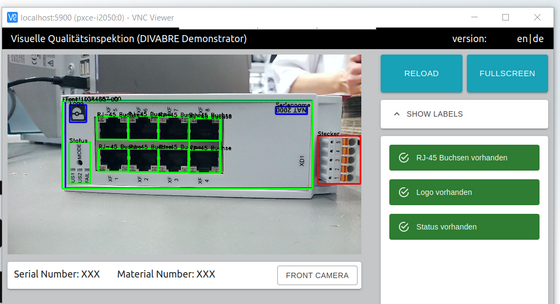
Visual inspection: Image-based methods for the automation of quality controls and the precise localisation of anomalies and damage.
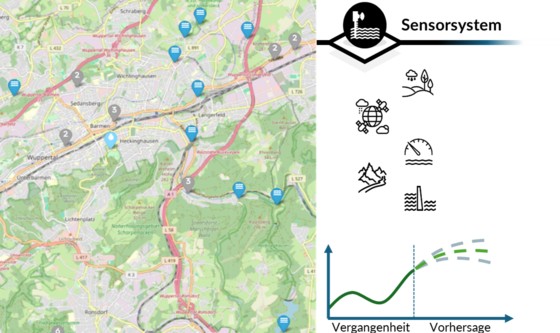
Sensor-based situation and condition assessment: processing and utilisation of transient and seasonal sensor data for condition monitoring, anomaly detection and forecasting.
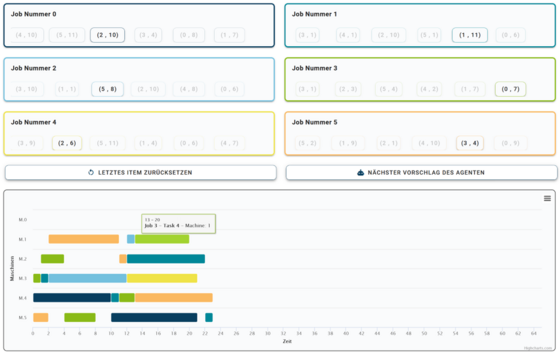
Intelligent planning and process design: Learning methods for solving complex planning and optimisation problems and for evaluating and parameterising processes.
Our research addresses a broad spectrum of deep learning technologies, including various learning paradigms such as supervised and reinforcement learning, learning scenarios such as transfer learning, representation learning and explainable AI, as well as model architectures such as transformer networks, autoencoders and generative adversarial networks.
We work closely with industry partners, whether as part of publicly funded projects or direct R&D contracts. In doing so, we deal intensively with real challenges and always take the needs of end users and technical experts into account. This practical orientation ensures that our research results are not only theoretically sound, but also directly applicable in industrial practice and improve value creation.
Selected publications
- 2024
- Saini, L., Su, Y., Tercan, H., & Meisen, T. (2024). "CenterPoint Transformer for BEV Object Detection with Automotive Radar" in Proceedings of the IEEE/CVF Conference on Computer Vision and Pattern Recognition (CVPR) Workshops . 4451—4460.
- Alves-Gomes, M., Meyes, R., Meisen, P., & Meisen, T. (2024). "It’s Not Always about Wide and Deep Models: Click-Through Rate Prediction with a Customer Behavior-Embedding Representation" , Journal of Theoretical and Applied Electronic Commerce Research , 19 (1), 135—151.
- Hütten, N., Alves-Gomes, M., Hölken, F., Andricevic, K., Meyes, R., & Meisen, T. (2024). "Deep Learning for Automated Visual Inspection in Manufacturing and Maintenance: A Survey of Open- Access Papers" , Applied System Innovation , 7 (1), 11.
- Waubert-de-Puiseau, C., Dörpelkus, C., Peters, J., Tercan, H., & Meisen, T. Beyond Training: Optimizing Reinforcement Learning Based Job Shop Scheduling Through Adaptive Action Sampling.
- 2024
- Alves-Gomes, M., Meyes, R., Meisen, P., & Meisen, T. (2024). "It's Not Always about Wide and Deep Models: Click-Through Rate Prediction with a Customer Behavior-Embedding Representation" , Journal of Theoretical and Applied Electronic Commerce Research , 19 (1), 135--151.