Constantin Waubert de Puiseau, M.Sc.
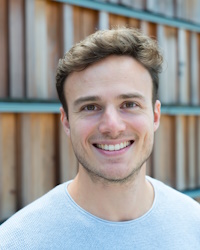
Research Associate
Research interests:
- Deep reinforcement learning for production planning and warehouse logistics
- Industrial Deep Learning and Data Science
- Artificial Intelligence in Operations Research
Biography
Constantin Waubert de Puiseau joined the Institute for Technologies and Management of Digital Transformation at the University of Wuppertal as a scientific researcher and Ph.D. student in December 2019.
Mr. Waubert de Puiseau studied mechanical engineering at RWTH Aachen University, with a focus on information technology applications in production and medical engineering. In his master thesis, he investigated artificial neural networks and developed methods to visualize the representation of knowledge stored in the networks.
At the TMDT he works on machine learning applications in the industrial context. A particular focus, which is the core of his dissertation, is research on robust and interpretable production scheduling using deep reinforcement learning.
Publications
- Waubert-de-Puiseau, C., Dörpelkus, C., Peters, J., Tercan, H., & Meisen, T. Beyond Training: Optimizing Reinforcement Learning Based Job Shop Scheduling Through Adaptive Action Sampling.
- 2023
- Waubert-de-Puiseau, C., Tercan, H., & Meisen, T. (2023). "Curriculum Learning in Job Shop Scheduling using Reinforcement Learning" .
- Waubert-de-Puiseau, C., Peters, J., Dörpelkus, C., Tercan, H., & Meisen, T. (2023). "schlably: A Python Framework for Deep Reinforcement Learning Based Scheduling Experiments" , arXiv arXiv:2301.04182 .
- 2022
- Waubert-de-Puiseau, C., Nanfack, D. T., Tercan, H., Löbbert-Plattfaut, J., & Meisen, T. (2022). "Dynamic Storage Location Assignment in Warehouses Using Deep Reinforcement Learning" , Technologies , 10 (6), 129.
- Waubert-de-Puiseau, C., Meyes, R., & Meisen, T. (2022). "On reliability of reinforcement learning based production scheduling systems: a comparative survey" , Journal of Intelligent Manufacturing , 33 (4), 911—927.